GPT Engineer:an AI-powered application builder that generates codebases from project descriptions.
About GPT-Engineer
GPT-Engineer: Your New AI Coding Assistant
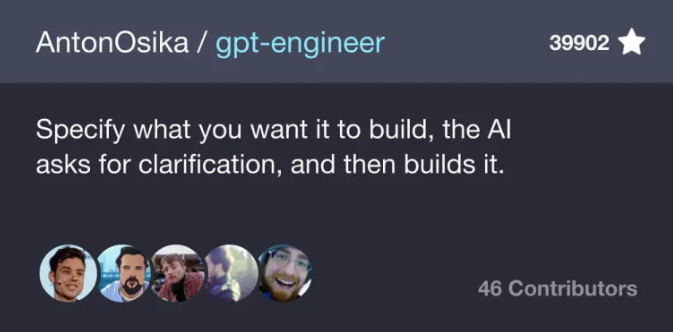
GPT-Engineer is an open-source agent that can help with code building. Simply describe what you want to build and the AI will ask clarifying questions before generating a full codebase. Key features include the ability to customize the AI’s coding style, improve generated code through feedback, and persist projects for later use. GTP-Engineer can be quite confusing to install and set up if you are not familiar with Python, we recommend carefully following the installation instructions found on the GitHub repository.
Specify what you want it to build, the AI asks for clarification, and then builds it.
GPT Engineer is made to be easy to adapt, extend, and make your agent learn how you want your code to look. It generates an entire codebase based on a prompt.
Setup
Choose either stable or development.
For stable release:
python -m pip install gpt-engineer
For development:
git clone https://github.com/AntonOsika/gpt-engineer.git
cd gpt-engineer
python -m pip install -e .
- (or:
make install && source venv/bin/activate
for a venv)
- (or:
API Key
Choose one of:
- Export env variable (you can add this to .bashrc so that you don’t have to do it each time you start the terminal)
export OPENAI_API_KEY=[your api key]
- .env file:
- Create a copy of
.env.template
named.env
- Add your OPENAI_API_KEY in .env
- Create a copy of
- Custom model:
- See docs, supports local model, azure, etc.
Usage
- Create an empty folder for your project
- If inside the repo, you can run:
cp -r projects/example/ projects/my-new-project
- If inside the repo, you can run:
- Create a file called
prompt
(no extension) and fill it with instructions gpt-engineer <project_dir>
- For example:
gpt-engineer projects/my-new-project
- For example:
By running gpt-engineer you agree to our terms.
Results
Check the generated files in projects/my-new-project/workspace
Workflow
gpt-engineer --help
lets you see all available options.
For example:
- To improve any existing project, use the flag:
-i
- To give feedback to/improve a gpt-engineer generated project, use:
--steps use_feedback
Features
You can specify the “identity” of the AI agent by editing the files in the preprompts
folder.
Editing the preprompts
, and evolving how you write the project prompt, is how you make the agent remember things between projects.
Each step in steps.py
will have its communication history with GPT4 stored in the logs folder, and can be rerun with scripts/rerun_edited_message_logs.py
.
You can also run with open source models, like WizardCoder.
Leveraging GPT-4 for Design Documentation Prompts
GPT-Engineer prompting is based on feeding it design documents. But how does one draft a useful design document? Why not use GPT-4 to help create them?!?
Let’s use GPT-4 to generate detailed project design descriptions, which can then be fed into GPT-Engineer. This way, you can ensure that your AI coding buddy has all the information it needs to build your application.
As an example, let’s create a design document with GPT-4 to prompt GPT-Engineer with. It really doesn’t take much to get ChatGPT to produce a useful design document — though they can be more complex, as you will see below. Here is our prompt:
I want to implement a game of snake in Python, using the game’s classic rules and gameplay. It can use external libraries to accomplish this. Generate a design document for this project.
Here are the first several lines of the generated design document, which I can assure you, in total, does a good job of outlining what needs to be done.
Introduction
The purpose of this design document is to outline the implementation details for creating a classic Snake game in Python. The game will adhere to the traditional rules and gameplay, where the player controls a snake that grows in length by eating food while avoiding collisions with itself and the game boundaries. The game will be developed using external libraries to facilitate graphics and user input.Game Components
The Snake game will consist of the following components:
a) Snake: A movable object that grows in length when it eats food and loses the game if it collides with itself or the game boundaries.
b) Food: A randomly placed object that the snake can eat to increase its length and score.
…
Now that we have a design document prompt, we can use it with GPT-Engineer.
Building a Design Document with ChatGPT
One of the great things about GPT-Engineer is its versatility. It can implement a simple snake game, just as it can help you build a wide range of applications, including a simple key-value database using only Python’s standard library modules. Just describe the project in the main.prompt
file, and GPT-Engineer will ask you for any necessary clarifications. Once you’ve provided the details, sit back and watch as GPT-Engineer generates your codebase.
First, let’s generate a design document with ChatGPT-4. Remember, you don’t need to use ChatGPT to accomplish this. If you have a detailed description of the software you are looking to build you are able to use it. But for a comprehensive conversion of your design notes to something that is easily ingestible by GPT-Engineer, this is a logical route to take.
Here is the prompt the author used with ChatGPT-4 to generate a design document:
Generate a high quality design document that can be used by our project team to design the software described below. It should be complete and usable as the sole design documentation to get our team to complete our project.
—
Simple key-value database engine written in Python using only standard library modules (except those mentioned below).The basic interaction with the database engine when it is running should be via imported Python module with a clear and concise library API.
There should also be a separate console that can be run to act as a command line REPL for interacting with the database engine and databases.
Additional methods of interaction should include an API created with Fast API (external library).
Design specifications:
A key-value database is a type of NoSQL database that stores data as a collection of key-value pairs. Unlike traditional relational databases, key-value databases do not enforce a rigid schema, providing flexibility and scalability for certain use cases. Designing a key-value database involves considering several important specifications.
Commands that should be accessible:
– connect to database
– delete database
– get key
– insert data
– update data
– delete data
– search for existence of both keys and valuesData Model: Each record consists of a unique key and an associated value. The value can be a simple scalar value or a complex data structure (such as JSON or XML).
Key Structure: The key serves as the primary identifier for each data entry and must be unique within the database. Keys can be alphanumeric strings, integers, or even composite keys (multiple values combined to form a single key).
Value Structure: The value associated with each key can be of any data type. It can range from basic data types (strings, numbers, booleans) to more complex structures like nested objects or arrays.
Performance and Caching: In-memory caching techniques should be employed to speed up read operations and reduce latency.
And here is the design document that ChatGPT-4 produced:
## Overview
The main goal of this project is to design and develop a lightweight key-value database engine written in Python, using only standard library modules, and FastAPI for the HTTP interface. The application will provide the essential features of a key-value database, including connecting to the database, data insertion, update, deletion, and retrieval, along with search functionality for keys and values.The database will be interfaced via three methods:
1. A Python module that can be imported by other Python programs.
2. A command line REPL console for manual interaction.
3. An API created with FastAPI for remote interactions.## Components
The database engine will have the following main components:1. **Data Store**: The actual storage for the key-value pairs. As a simple Python implementation, this could be a dictionary where keys are unique identifiers and values can be of any datatype. The data store should have efficient methods for accessing, adding, updating, and deleting data.
2. **Database Engine**: The engine that provides the methods to interact with the data store. This will include methods to connect to a database, insert, update, and delete data, as well as fetch a value by key. It should also include a method to check the existence of a particular key or value in the data store.
3. **In-Memory Cache**: A cache layer that holds frequently accessed data to improve read speed and reduce latency. This can be implemented using Python’s `functools.lru_cache` decorator or a custom caching solution.
4. **CLI**: A command-line interface for manual interaction with the database engine. This would use the `cmd` or `argparse` module from the Python standard library to create a REPL interface.
5. **HTTP API**: A FastAPI application that exposes the database engine methods as a HTTP API. This will allow remote interaction with the database.## Data Model
The database engine will follow a simple key-value data model. Each record in the database will consist of a unique key and an associated value.### Key Structure
Keys can be alphanumeric strings, integers, or composite keys. They must be unique within the database and will serve as the primary identifier for each data entry.### Value Structure
The value associated with each key can be of any Python data type. It can range from basic data types (strings, numbers, booleans) to more complex structures like nested objects or arrays.## API Design
The Python library API, CLI, and FastAPI should provide the following functions:
– `connect(database_name: str)`: Connect to a database. Creates the database if it does not exist.
– `delete_database(database_name: str)`: Deletes a database.
– `insert(key: str, value: Any)`: Inserts a new key-value pair into the database.
– `update(key: str, value: Any)`: Updates the value of an existing key.
– `delete(key: str)`: Deletes a key-value pair from the database.
– `get(key: str)`: Fetches the value of a key from the database.
– `exists_key(key: str)`: Checks if a key exists in the database.
– `exists_value(value: Any)`: Checks if a value exists in the database.## Performance and Caching
In-memory caching techniques will be employed to speed up read operations and reduce latency. The `functools.lru_cache` decorator or a custom caching solution could be used for this purpose. The cache should have a maximum size to prevent excessive memory usage.## Conclusion
This document provides a high-level design for a simple key-value database engine using Python and FastAPI. By adhering to this design, we can ensure that the database will be flexible and scalable, meeting the needs of various applications.
And that’s it. The only thing left to do was run GPT-Engineer and direct it to these criteria.
How To Install GPT Engineer
This gpt-engineer installation guide will walk you through the process of installing GPT Engineer on Windows PC from official GitHub repository. First, we’ll need to install Git and Python. Optionally you can install VSCode, but you can use any favorite IDE or text editor.
FAQ
What is GPT engineering?
GPT-Engineer is an AI-powered application builder that generates codebases from project descriptions. It simplifies building applications, including our key-value database example, and works well with GPT-4.
Is GPT Engineer free?
GPT Engineer is used for generating a complete codebase in any computer language from a text prompt description. It’s available for free from official GPT Engineer GitHub repository. Note, that GPT Engineer requires an OpenAI GPT 4 API key. Every time you use it, you will be using your GPT 4 tokens.
Is GPT Engineer only for Python?
Set Up Python Environment
GPT Engineer requires Python to run.
What is the difference between AutoGPT and GPT Engineer?
Comparing gpt-engineer and AutoGPT
While AutoGPT is a powerful tool in its own right, gpt-engineer offers a more specialized and focused approach to code generation and technical specification.